The OEE Illusion: Why "Fuzzy Math" Hinders True Manufacturing Improvement
- Matt Ulepic
- Mar 27
- 6 min read
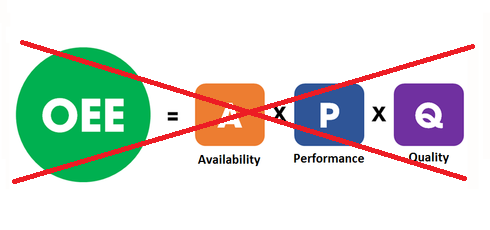
For decades, Overall Equipment Effectiveness (OEE) has reigned as a key performance indicator (KPI) in the manufacturing world. It's often presented as the gold standard for measuring how effectively a manufacturing operation is being utilized relative to its full potential. The allure is undeniable: a single, comprehensive metric that encapsulates availability, performance, and quality. It’s the promise of a clear, concise snapshot of operational efficiency.
However, beneath the surface of this seemingly straightforward calculation lies a complex web of subjectivity, data integrity issues, and a fundamental disconnect from the granular realities of the shop floor. In my experience, OEE often devolves into what I call "fuzzy math" – a metric susceptible to manipulation, prone to inaccuracies, and ultimately, a less-than-reliable guide for genuine continuous improvement.
Think about it. Walk into different manufacturing facilities, and while they might all claim to calculate OEE, you'll likely find subtle, and sometimes not-so-subtle, variations in their methodologies. It's akin to different accounting practices – perfectly legal, perhaps, but capable of presenting vastly different financial pictures to suit a particular audience or narrative. This inherent flexibility undermines the very purpose of a standardized metric, making true benchmarking and cross-industry comparisons a dubious exercise.
The inherent fuzziness stems from the very variables that constitute OEE: Availability, Performance, and Quality. While seemingly objective on the surface, each of these components is often deeply intertwined with human interpretation and subjective nuances that can be easily leveraged to justify either favorable or unfavorable results.
Availability: The Human Element of Downtime
Availability, typically calculated as the ratio of actual uptime to planned production time, should theoretically be a straightforward measure of whether equipment is ready to run when it's supposed to. Yet, the human element injects significant variability. Consider the scenario I often encounter: an operator is unexpectedly absent during the first shift. To compensate, they work extra hours during the subsequent shift. While the total production hours for the day might appear reasonable, the OEE calculation for that initial shift will undoubtedly plummet due to the unplanned downtime.
Is this a true reflection of the machine's inherent availability? Or is it a consequence of staffing logistics? The OEE metric, in this instance, penalizes the shift for a human resource issue, potentially masking other underlying problems or creating a skewed perception of the machine's reliability. Similarly, decisions about planned maintenance schedules, minor stoppages that may or may not be meticulously recorded, and even the definition of "planned production time" itself can be subject to interpretation, subtly influencing the availability figure.
Performance: The Shifting Sands of Speed
Performance, usually defined as the ratio of actual production speed to ideal production speed, aims to capture whether the equipment is running at its optimal rate when it is available. However, the "ideal" rate itself can be a moving target. I've heard countless justifications for performance deviations: "We had some material inconsistencies this week, so we had to run the machine slower to maintain quality." Or, conversely, "Our most experienced operator was on this line, and they consistently push the machine a bit faster than the standard."
While these explanations might hold some validity, they introduce a significant degree of subjectivity into the performance calculation. What constitutes a "machine issue" that warrants a slower speed? How consistently is the "ideal" rate actually achievable under normal operating conditions? The performance metric, in these cases, becomes a reflection of transient factors – material quality, operator skill – rather than a stable measure of the equipment's inherent capability. This makes it challenging to identify true bottlenecks or areas where the machine itself could be optimized for better throughput.
Quality: The Trainee Effect and Beyond
Quality, typically measured as the ratio of good units produced to the total units produced, is perhaps the most directly influenced by human factors. The example of a new operator undergoing training is a classic illustration. During their learning curve, it's almost inevitable that scrap rates will be higher, leading to a dip in the quality component of OEE.
While tracking quality is crucial, attributing a temporary dip solely to operator training can obscure other potential quality issues, such as inconsistent raw materials, poorly maintained tooling, or inadequate machine setup procedures. The OEE metric, in this scenario, might simply highlight the presence of a new employee rather than pinpointing systemic problems that need addressing. Furthermore, the definition of a "good unit" can sometimes be subject to interpretation, particularly in complex manufacturing processes with varying levels of acceptable defects.
The Tyranny of Multiplication: Small Volatility, Big Impact
The fundamental flaw in the OEE calculation lies in the fact that these three often-subjective variables – Availability, Performance, and Quality – are multiplied together to arrive at a single percentage. This multiplicative effect means that even slight volatility in any one of the components can have a dramatic impact on the overall OEE score. A minor dip in availability due to a brief stoppage, coupled with a slight reduction in performance due to a minor machine adjustment, and a small increase in scrap from a temporary issue can collectively lead to a significant and potentially misleading drop in OEE.
This volatility makes it difficult to discern the true underlying causes of inefficiency. Is the low OEE a result of frequent small stoppages, consistently suboptimal speeds, or occasional quality spikes? The single OEE number provides little granular insight into where the real opportunities for improvement lie.
The Data Dilemma: The Perils of Manual Entry
Beyond the inherent subjectivity of the OEE components, the reliability of the data used in the calculation is another significant concern. In far too many manufacturing environments, the values for uptime, downtime, production counts, and scrap rates are still captured manually by operators. While the intention is often good, manual data entry is notoriously prone to errors – missed entries, inaccurate recordings, and even intentional or unintentional biases can creep into the data.
Imagine an operator under pressure to meet production targets. They might be less inclined to meticulously record every minor stoppage, potentially inflating the availability figure. Similarly, estimates of production speed or counts can be inaccurate, skewing the performance metric. This reliance on manual data undermines the very foundation of OEE, rendering it a potentially flawed representation of actual operational performance.
The Hawthorne Effect: Performance Under the Microscope
Finally, the context in which OEE-related data is often collected can further distort the picture. When time studies are conducted, or during Kaizen events focused on improving OEE, there's an inherent "Hawthorne effect" at play. Knowing they are being observed, operators and maintenance personnel are naturally inclined to be on their best behavior. Machines might receive extra attention, processes might be followed more diligently, and overall performance might temporarily improve.
The data collected during these periods, while valuable for identifying potential improvements under ideal conditions, seldom reflects the true day-to-day reality when the spotlight is off. This skewed data can lead continuous improvement (CI) teams to focus their efforts on addressing issues that are only apparent under scrutiny, potentially overlooking the more pervasive and impactful inefficiencies that occur during normal operations.
The MachineTracking Approach: Objective Clarity Over Fuzzy Metrics
It is precisely these inherent limitations and potential for misinterpretation that led us at MachineTracking to take a different approach. We recognized that chasing the often-volatile and potentially misleading OEE metric could distract manufacturers from identifying the true drivers of lost productivity.
Instead of relying on subjective inputs and manual data collection, MachineTracking focuses on capturing a single, objective metric: making-chip time. Our system directly monitors machine activity in the background, automatically and without any human involvement. This provides a genuine, unbiased record of when the machine is actively engaged in production.
This objective data offers a far clearer and more reliable picture of actual productivity. It eliminates the subjective nuances of availability, the fluctuating interpretations of performance, and the human-influenced variations in quality. When a machine isn't making chips, our data clearly identifies the periods of inactivity, allowing manufacturers to pinpoint exactly when and where productivity is not taking place as expected.
This objective approach fosters more meaningful conversations with your team. Instead of debating the validity of OEE figures or trying to decipher the underlying causes of a low score, you can focus on the concrete data of when the machine was idle. This facilitates targeted problem-solving and allows your continuous improvement efforts to be directed towards the real opportunities for enhancing efficiency.
Stop Chasing the OEE Illusion: Embrace Objective Uptime Tracking
While OEE holds the promise of a comprehensive performance metric, its inherent reliance on subjective inputs, error-prone manual data collection, and susceptibility to situational biases often renders it a "fuzzy math" exercise that can obscure the true picture of manufacturing efficiency.
Instead of wasting valuable time and resources on complex OEE calculations and potentially misleading data collection efforts, it's time for manufacturers to embrace a simpler, more objective approach. By focusing on automatically tracking genuine making-chip time, as MachineTracking does, you can gain a clear, unbiased understanding of your actual productivity, empower data-driven decision-making, and direct your continuous improvement initiatives towards the areas that will yield the most significant and sustainable results. Stop chasing the OEE illusion and start focusing on the objective truth of your machine's uptime. Your bottom line will thank you.